The financial sector is increasingly using machine learning in order to be more accessible, efficient and provide a better customer experience overall.
In fact, in a recent survey carried out by the Financial Conduct Authority and the Bank of England (BoE) almost two-thirds of financial service companies surveyed said they used machine learning.
But in what ways exactly are machine learning models implemented? It is used in a number of key applications and can be used to help with a range of services, from providing advice to even minimising risk, but how? We decided to take a closer look.
What is machine learning?
To clarify, machine learning is a type of methodology where the algorithm effectively makes decisions with limited or in some cases, no human intervention at all.
How is machine learning different from artificial intelligence?
Machine learning often ends up getting confused with artificial intelligence, but the two are different from each other, and it is important to know the difference.

Artificial intelligence is the theory as well as the development of computer systems that perform tasks that previously needed a human to do it. AI for Banking works differently. Machine learning is a sub-category of artificial intelligence.
Anti-money laundering
Machine learning algorithms are being used by anti-money laundering teams in the finance sector to help tackle criminals. Machine learning models make it much easier (and with greater accuracy) to detect those committing a crime.
Robo investment advisors
Another way in which the finance industry is using machine learning to streamline their services is through robo advisors. For example, there are now a number of automated investment service apps available on smartphones that allow people to access excellent advice on making strategic investment decisions, as well as being able to improve their financial health. The machine learning capacities of the software mean that it can identify the best opportunities or strategies available and as it grows, the model becomes smarter due to the growing amounts of data it accumulates.
Customer support
Similar to robo advisors are the common occurrence of chatbots amongst banks and other financial service providers, that interact with customers to ultimately provide great customer service. Every company worth its weight understands just how vitally important customer service is to the longevity of the firm and building its reputation, which in turn improve profits.
Machine learning can help with this through a variety of applications, and over time better decides the right response to give to a customer so as to not aggravate them.
These models can also identify the best way of contacting a customer regarding an issue, which can help to reduce potential problems (such as irritating a customer) at a later date.
Risk management
Machine learning is also being used to lower the risk poised to financial institutions. How is this being achieved? Let's take a look at an example. If a bank is trying to determine whether to approve someones loan application, they can use web data integration to analyse extremely high volumes of personal information to mitigate their risk.
This can make it far easier for a bank to decide whether to accept or decline their loan application, compared to simply having to make a decision based on the applicant's credit score.
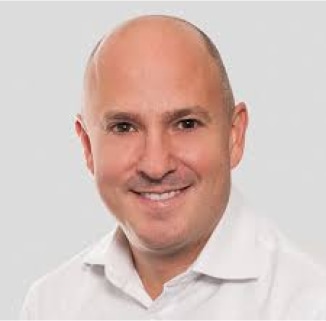
Dani’s actuarial experience and passion are key. He is a strong advocate of innovation, optimism and communication, both within the team and for the clients. Dani’s ability and experience with data ensure that we always maximise value and efficiency for every project, enabling us to unlock hidden value for the clients business.