Clarity Through Separation: Why Splitting Frequency and Severity Works
Building predictive models to determine the technical premium of a commercial insurance contract is not a trivial task. The Frequency-Severity(FS) model is one possible approach to this problem and in the world of machine learning (ML) is still a popular solution often chosen as a good balance between predictability and explainability when utilising GLMs.
Our pricing expert, Karol Gawlowski has shared some of his thoughts on the essential considerations when building FS models and underlines why commercial insurance might be a more challenging environment than personal insurance in this context.

Start with the Data: The Non-Negotiable First Step
At a basic level, breaking down losses into claim frequency and claim severity helps actuaries and underwriters gain a clearer view of the risk at hand. This dual approach opens the door to a better understanding of the risk drivers as compared to the pure premium or ML approach, where model parameters are either obfuscated or do not uncover all identifiable patterns from the data. Put simply, a pure premium model does not allow us to easily distinguish between risks that might generate many low-severity claims from the opposite – infrequent but large losses which might increase the book’s overall volatility.
Having that knowledge might also be beneficial when considering contracts that attach at different points in the tower. In that context, despite the availability of interpretability techniques such as SHAP, ML methods remain significantly less popular.
Before we go further, it must be emphasized that, as with all modelling exercises, the first step—data collection, cleaning, and preparation—is the most important, as noted in last month’s release on AI and ML.
Why Commercial Insurance Is a Different Beast
The ability to identify low‐frequency, high‐severity risks is far more crucial in the commercial space than in personal lines, given the sheer size of written exposure and the likelihood of a large claim.
There are many other pain points when drawing the comparison between commercial and personal lines – commercial data usually suffers from much more heterogeneity, and there’s also much less volume when it comes to the number of model points. For instance, in a fairly undeveloped Employers' Liability book, even within the same SIC code, we might find records with strikingly different risk profiles, and there is insufficient data volume to derive concrete, meaningful patterns that distinguish them from random variation. le to build a model with high granularity – for example, modeling at a vehicle level vs for an entire fleet in Commercial Auto. Comparing these two scenarios, the former is likely to offer much deeper insight into an account’s risk quality by highlighting, for example, vehicles or drivers’ profiles that fall outside an insurer’s appetite.
Not to mention encountering missing exposure data, which raises the question of whether to discard the faulty entries, thereby further reducing the data volume, or to try various imputation strategies. Similarly, writing risks at specific layers will often result in left and right censoring of claim amounts, providing the insurer with only partial information about a claim when it breaches the attachment point.
The Art and Science of Variable Selection
Variable selection is also challenging because it is not purely a quantitative exercise and requires incorporating underwriters’ views. Although there are techniques that could potentially help with this (such like GLMs derived using proximal regularisation) they might increase the complexity of technical discussions and the stakeholder buy-in.
When Should You Use an FS Model?
Modelers and portfolio managers have to be mindful that not every LoB is suitable for rolling out an elaborate stochastic pricing model. Even if the data systems are in place and data quality is satisfactory, that does not necessarily mean that making the leap from a rating table to a complex pricing engine is an optimal decision.
All models should be compared and tested out of sample and any increase in their complexity must be justified by a reasonable improvement in predictive performance.
In other words, we are paying for the ability to better price and select risk by trading off the time needed for LoB actuaries and underwriters involved to become fully comfortable with the new tool.
What Models Do Best: Augmenting Human Judgment
These obstacles do not imply that FS or any other modelling efforts should be entirely abandoned. The model fitting can be more mathematically and technically challenging, and may require more manual adjustments from underwriters, but ultimately, it is impossible for a human brain to fully comprehend and remember the performance of an entire portfolio and to make unbiased decisions based solely on one’s memory. This is the role of a model –to distill these patterns and provide underwriters with a compressed and accurate historical indication of the portfolio’s performance.
Built by Actuaries, for Actuaries: The Optalitix Edge
Optalitix isn’t the only pricing software out there, but it has been created and developed by a Corporate Actuary who understands the challenges first-hand. Therefore, the Optalitix platform includes advanced no-code solutions which are fast to implement, easy to adopt,and address user needs. We would welcome the opportunity to chat with actuaries who are interested in exploring their options.
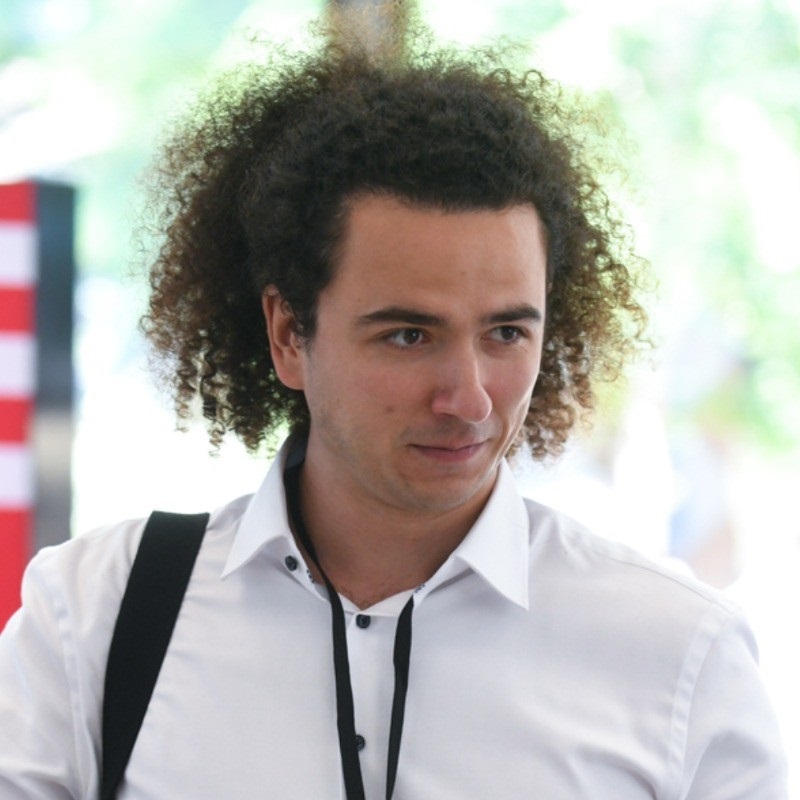
Karol Gawlowski is the Chair of the IFoA’s Actuarial Data Science Working Party and a Predictive Modeler at Allianz Commercial. He holds two MSc degrees—one in Actuarial Science with Business Analytics from Bayes Business School and another in Quantitative Methods from the Warsaw School of Economics—and has guest lectured on Python programming for MSc students. Karol is a frequent speaker at actuarial conferences, including GIRO, where he has presented on machine learning, explainable AI, and actuarial data science.